A common saying in the longevity world
How do you live to be 90?
Eat well.
Exercise.
Sleep.
Avoid alcohol.
How do you live to be 100?
Have parents that lived to be 100.
This speaks to an underlying biological truth - extending lifespan is not just about optimizing healthspan. We likely all know someone who was extremely healthy for their age until they, within a short period of time, died from a surprise illness. The aging vector that ultimately kills you is largely separate from the one that keeps you free from being sick.
A Tale of Two Mice
The mice on the top panel are regular mice at 22 months of ago. The mice on the bottom panel have had all of their senescent cells1 cleared. You’ll observe how much healthier the mice in the bottom panel are, and on average they lived about 25% longer.
But if you look at when the last mouse in the bottom panel died vs. when the last mouse in the top panel died2?
There’s barely a difference:
The average lifespan is increased, likely because removing the senescent cells prevents the mice from getting diseases that would kill them early (such as cancer). But the inevitable march towards death is not slowed, and the luckiest mice from the untreated cohort and the luckiest mice from the treated cohort die at about the same time.
Are there longevity interventions that extend maximum lifespan significantly?
There aren’t many. The clear standout is caloric restriction:
Here, you can see very clearly the last caloric-restricted mouse is dying much later than the mouse eating ad libitum. Their rate of aging is slower, not just their rate of accumulating diseases.
The difference between healthspan and maximum lifespan is not always so clear. If you treat mice with acarbose and rapamycin at 16 months of age, you do see both maximum and median lifespan extension… but the maximum lifespan extension is only readily apparent in females.
Some interventions primarily target healthspan; others primarily maximum lifespan. Some are a combination of both. But these two aspects of aging are distinct.
Is this true in humans?
A Tale of Two Clocks
In 2013, Steve Horvath released his epigenetic clock paper demonstrating that you could train a machine learning model to predict age to within about three years (more on this here). This was a fascinating result, but a number of folks found the idea of being able to predict your age underwhelming - after all, everyone has a sense of how old they are3.
This led to the generation of second-generation epigenetic clocks, which were created to predict health as opposed to age. Two different approaches led to two very different clocks:
Morgan Levine’s PhenoAge, which was trained to predict health markers alone and then regressed onto age.
Ake Lu’s GrimAge, which was trained to predict time-to-mortality.
If health and mortality were the same, then the two clocks should be redundant as their predictions would be the same. The data show the opposite. Here is a plot showing their correlations:
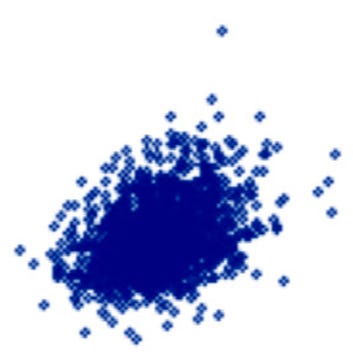
Quantitative estimates vary, but the correlation here is mediocre, with an R of somewhere between .3 and .5. There is not perfect disentangelment here - a clock trained on mortality data will pick up a lot of healthspan markers, as humans generally do not live to their absolute maximum lifespan potential. In fact, GrimAge was initially built from a composite of healthspan markers that, together, predict mortality. Nevertheless, it’s interesting that these two measures diverge.
Human genetics studies show a similar pattern:
Genetic variants that are linked to extreme longevity are not the same ones that are linked to healthspan. In fact, some genetic variants are connected to improved lifespan but worsened healthspan.
A Tale of Two Worms
For people working on aging research in C. elegans, this concept is not new at all (excellent paper on this topic here). Consider the well-known daf-2 mutant, which nearly doubles worm lifespan:
The effect on worm movement, however, is mostly negative - even at young worm ages.
Not every maximum lifespan-benefiting intervention has this tradeoff. Caloric restriction, which I mentioned earlier as having a major effect on maximum lifespan, seems to generally improve health metrics too (though not if taken to an extreme). But it’s an important parameter to keep in mind.
So what’s the take?
I once made a poorly-received joke to the XPRIZE Healthspan4 competition organizers that I would be interested in proposing giving cocaine and steroids to the elderly in order to reverse their age. Given the therapeutic treatment window only lasts a year, this should be a clear winner!
The deep take is this: maximum lifespan and healthspan are separate; what is good for one may be useless or even detrimental for the other. Beware claims of reversing aging - depending on which aspect of aging is being measured, this may be a Faustian bargain trading health for time. For preclinical and clinical trials focused on aging, it will become increasingly important to ensure that we know whether a therapeutic is improving healthspan, lifespan, or both.
Further Reading
I kept this piece relatively light on aging theory. For folks interested in reading on this more deeply, I’d heavily recommend reading some of the work done by Peter Fedichev and Uri Alon. Physicists, for whichever reason, have picked up on the healthspan vs. maximum lifespan separation most acutely - perhaps it’s because they’re most attuned to the shape of the lifespan curves I shared in the beginning of the piece.
Senescent cells (sometimes referred to as “zombie cells”) are cells that stop dividing and become problematic, mostly via spitting out pro-inflammatory proteins. They accumulate with age in mice and likely humans. Killing off senescent cells was a very popular topic in the longevity world for a time.
A definition of “maximum lifespan.”
If I had a nickel for every time I heard the “if you want to predict your age, just check your driver’s license” snarky remark…
A $100M competition to find therapeutics, in people, that reverse aging by seven years within a one-year window.
Thank you for an excellent overview and commentary. Any chance of more details for the Ying et al 2024 ref for the forest plot? I tried 3 Ying 2024 articles and failed to find this interesting figure.